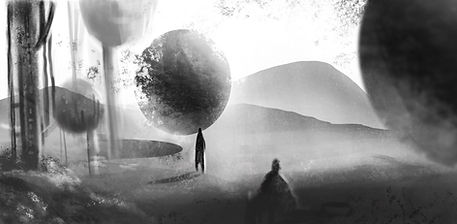

Nosework dogs have important roles in our everyday safety and security, from search and rescue to bomb detection. However, it takes many years for a dog handler to learn to recognize and understand the subtle cues from their canine partner. In this pilot study, we try to bridge the communication gap between nosework dogs and their handlers by developing a wearable device for dogs that collects audio and IMU data fed into machine learning algorithms to classify and predict nosework behaviors. The wearable device we developed uses a cardioid mic and two IMU sensors with the following design goals in mind: (1) dog comfort (2) ease of use for the handler and (3) reducing noise and issues due to the environment. We collected and labeled 20 minutes of audio and IMU data from a nosework dog performing searches while wearing our sensors. In this preliminary phase of the study, we focused our analysis on the audio data only, and we were able to classify sniffing audio events (idle vs sniffing) with 94.4% accuracy, which is a promising start for future work in this area. Our hope is that this pilot study will provide a proof of concept for more advanced systems that can provide finer granularity of behavior that may, for instance, take into account the sniffing frequency and sequence of movement patterns from the IMU sensors in order to better understand the changes in the dog's behavior while searching.